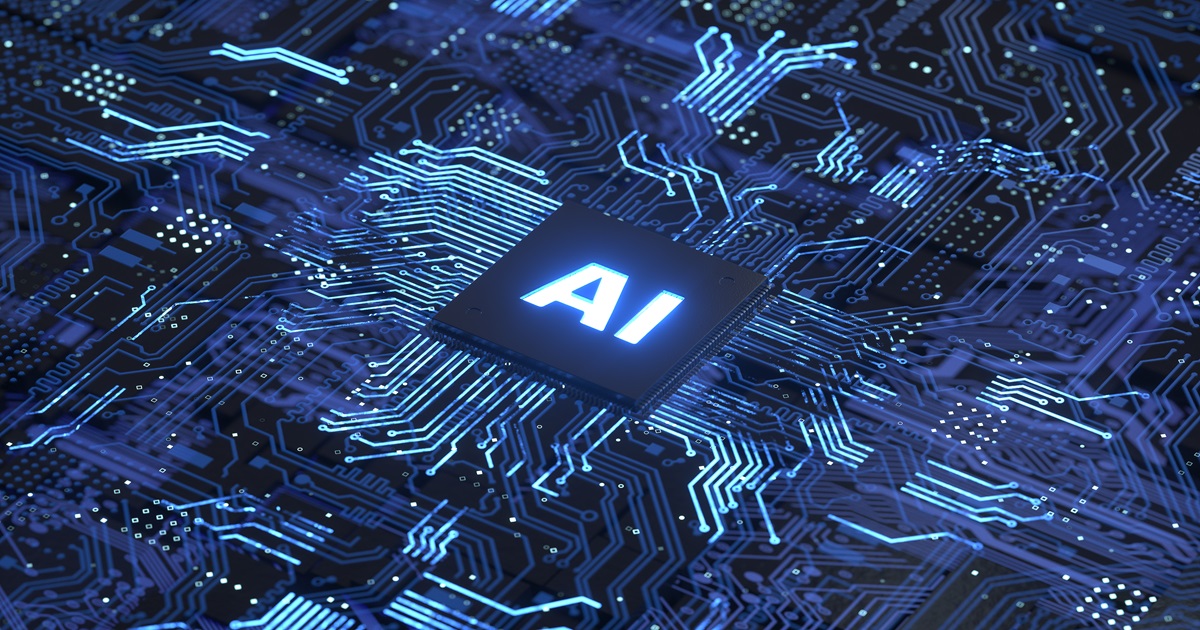
Businesses are pouring money into generative AI, but unlocking its full potential requires wrestling with a messy monster: unstructured data. This data, often containing sensitive customer and business information, sits locked away in various formats across different systems. It's estimated that 90% of enterprise data falls into this category, with organizations generating 73 exabytes of it in 2023 alone, according to an IDC report.
The catch? Extracting and standardizing this data is a time-consuming nightmare for developers. It's like sifting through a mountain of gold nuggets mixed with gravel. A 2023 IDC survey revealed that half of companies have their unstructured data trapped in isolated silos, and 40% still resort to manual extraction – a process akin to panning for gold with a teaspoon.
Data privacy adds another layer of complexity, especially when using outside AI models. Nearly half of companies surveyed by IDC cited compliance as a major hurdle. The fear? Sensitive information leaking out or being inadvertently memorized by the AI, which leads to hefty fines. Organizations must tread carefully to unlock the value of their data without compromising privacy.
In a recent move to eliminate data integration and data privacy challenges that hinder enterprise adoption of generative AI, Tonic.ai, the San Francisco-based company pioneering data synthesis solutions for software and AI developers, launched a secure data lakehouse for LLMs, Tonic Textual.
Tonic Textual is an all-in-one data platform designed to eliminate integration and privacy challenges ahead of Retrieval-Augmented Generation, or RAG, ingestion or LLM training — two of the biggest bottlenecks hindering enterprise AI adoption.
With Tonic Textual, users build, schedule and automate data pipelines that efficiently extract and transform information from various file formats, including text documents, images and spreadsheets. This standardized data is then readily usable for embedding, ingestion into vector databases or fine-tuning LLMs.
Tonic Textual also prioritizes data security. The platform's built-in named entity recognition models, trained on extensive datasets, automatically identify and classify sensitive information within unstructured data. Users have the option to redact this information while preserving its semantic meaning with synthetic data. Doing this will make data privacy certain without sacrificing the value of the information for AI development.
To further enhance the functionalities of AI systems, Textual enriches vector databases with document metadata and contextual entity tags. This improves retrieval speed and ensures the relevance of information used by RAG systems.
Tonic Textual is committed to continuous development. The roadmap includes features that will further simplify the creation of generative AI systems built on proprietary data. These features prioritize balancing privacy and utility:
- Native integrations with popular embedding models, vector databases and developer platforms will create automated data pipelines for AI systems.
- Enhanced data management capabilities such as cataloging, classification, quality control, privacy reporting and access management will ensure responsible use of generative AI.
- An expanded connector library will allow AI systems to access data across various sources, including cloud data lakes, object storage, file-sharing platforms and enterprise applications.
The long-story-short of this? Utilizing its expertise in data management and realistic synthesis, Tonic.ai developed a solution to tame and protect siloed, messy and complex unstructured data into AI-ready formats ahead of embedding, fine-tuning or vector database ingestion.
“We’ve heard time and again from our enterprise customers that building scalable, secure unstructured data pipelines is a major blocker to releasing generative AI applications into production,” said Adam Kamor, co-founder and Head of Engineering, Tonic.ai. “Textual is specifically architected to meet the complexity, scale and privacy demands of enterprise unstructured data and allows developers to spend more time on data science and less on data preparation, securely.”
Edited by
Alex Passett