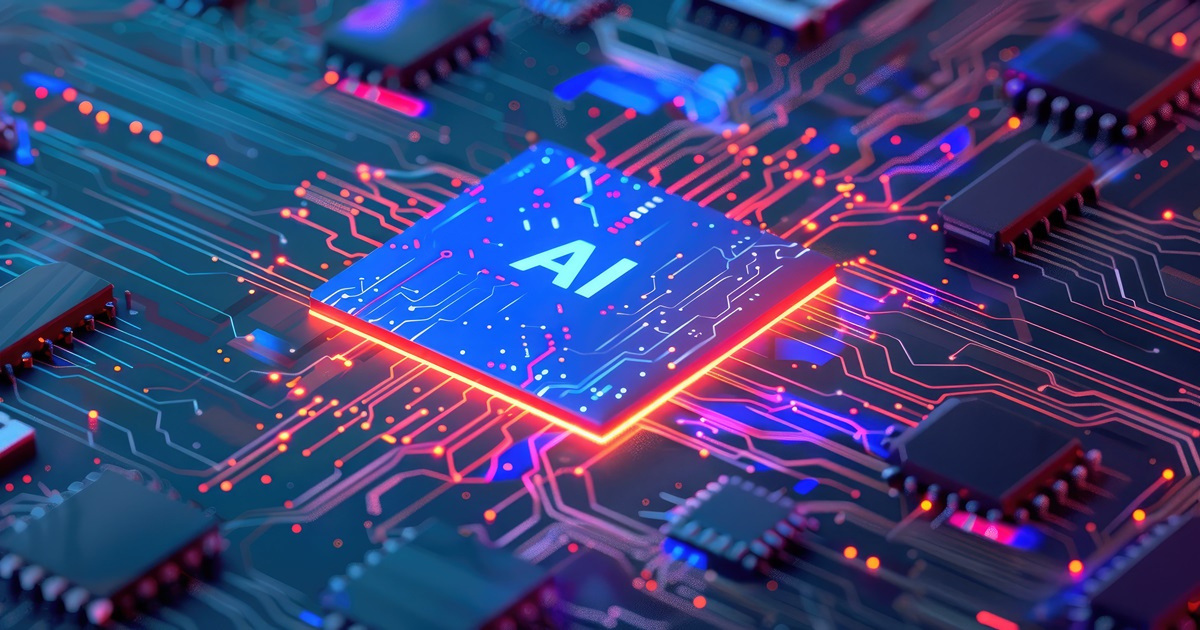
The democratization of AI development, fueled by open-source tools and resources, has lowered the barrier to entry for organizations of all sizes. That said, this accessibility masks the difficult road that lies ahead in the journey from research to production.
Despite the proliferation of AI models, a critical gap still persists between theoretical potential and real-world impact. The majority of these models fail to smoothly transition into production environments.
As mentioned, the path to production is fraught with obstacles, both technical and regulatory. Model blind spots, where the model's predictions are unreliable or biased, pose a threat to the integrity of AI systems. Additionally, the emerging landscape of AI regulations adds another layer of complexity, which demands that models be not only accurate but also compliant with a growing set of ethical and legal standards.
To address these challenges, LatticeFlow AI introduced its Suite 2.0 and presented the concept of The Last Mile of AI, a framework for the critical, final steps to ensure successful AI deployments.
LatticeFlow AI enables organizations to build performant, trustworthy and compliant AI applications. The Last Mile of AI encompasses five essential challenges: validate data, accelerate model performance, automate internal AI controls, achieve regulatory compliance, and monitor ongoing risks and performance.
With its Suite 2.0, LatticeFlow AI guides organizations through this “last mile” by enabling them to deliver AI systems that are not only technically performant, but also safe and compliant.
A core feature of Suite 2.0 is LatticeFlow AI's Health Checks, a capability that enables organizations to establish and automate internal controls for AI model performance and data quality.
Health Checks allow teams to standardize quality evaluations across their AI models tailored to each company’s needs. The suite contains a library of pre-defined checks that can be customized to the use cases at hand and offers the ability to create custom checks relevant for a company’s domain.
Health Checks operate on both data and model levels, as well:
Data Health Checks look into datasets and identify potential pitfalls like duplicate images, leaked samples or mislabeled data. This proactive approach prevents costly model errors and accelerates data preparation.
Model Health Checks, on the other hand, proactively detect risks such as model underperformance for specific metadata. Doing this ensures standardized diagnostics for different model versions.
Jumio, a provider of AI-powered identity verification and compliance solutions, has joined forces with LatticeFlow AI to utilize the strength of automated Health Checks. Jumio aims to proactively identify potential risks and enhance the security of its AI models. This partnership is about improving performance, staying ahead of industry regulations and ensuring compliance with emerging AI standards.
The long-story-short version here, readers?
Health Checks enable executives to have full confidence in the reliability and trustworthiness of their AI initiatives.
“We are at a pivotal time in AI, where safety, security, and compliance are no longer optional,” said Dr. Petar Tsankov, CEO and co-founder of LatticeFlow AI. “LatticeFlow AI is leading this evolution by automating key AI quality and risk controls, empowering teams to confidently navigate the last mile of AI and ensuring their AI applications are high-performing, trustworthy and safe for society.”
Edited by
Alex Passett